Webinaire TRACCS Janvier 2025 :
Combining physics and machine learning
in hybrid climate models
Publié le
📅 Vendredi 31 janvier de 11h à 12h
📍 Désormais disponible en replay : https://youtu.be/P7iX5Nn1Mok
Pour proposer d’intervenir lors du prochain webinaire, contactez contacts-traccs@listes.ipsl.fr
Chaque mois, TRACCS organise un webinaire afin de partager questionnements, controverses, idées et résultats scientifiques.
TRACCS vous donne rendez-vous vendredi 31 janvier de 11h à 12h pour son prochain webinaire « Combining physics and machine learning in hybrid climate models », présenté par Julien Le Sommer (CNRS).
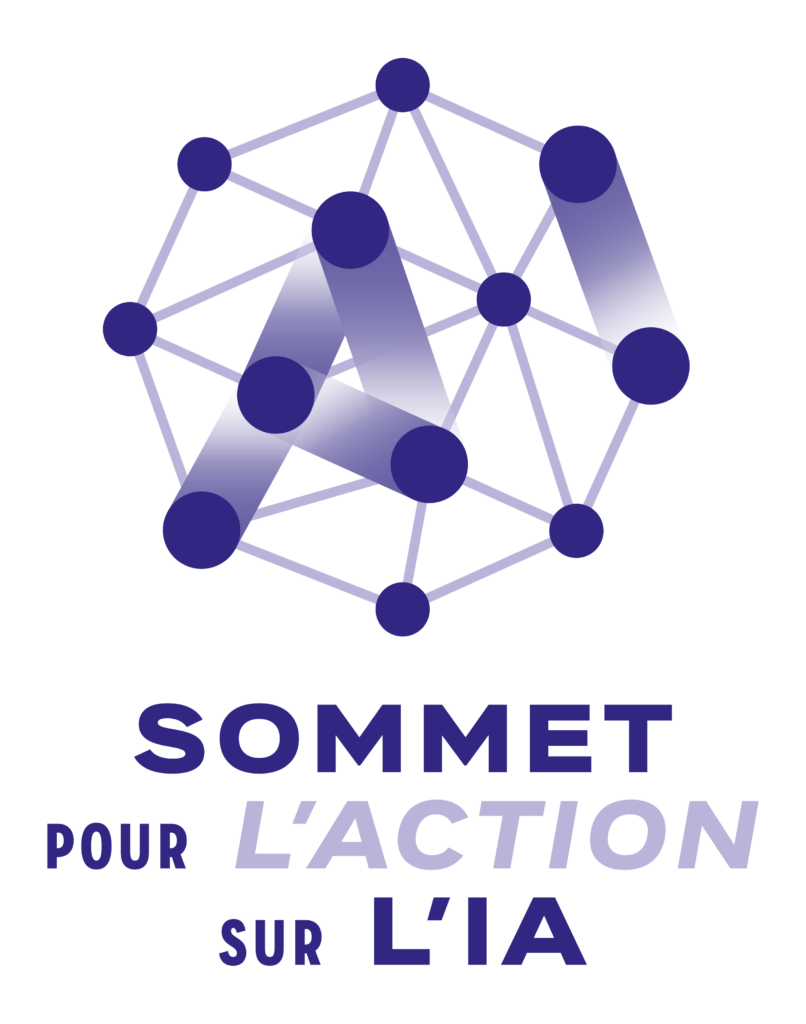
Ce webinaire porte sur les thématiques du projet ciblé 5 COMPACT de TRACCS. La réunion annuelle du PC5 et l’assemblée générale 2025 de TRACCS (4 au 6 février à Grenoble) ont été labellisées dans le cadre du Sommet pour l’action sur l’Intelligence Artificielle visant à renforcer l’action internationale en faveur d’une intelligence artificielle au service de l’intérêt général !
In recent years, scientific machine learning has emerged as a transformative approach in climate science and geoscientific modeling. Among its most impactful applications are emulators for weather forecasting, which now rival traditional physics-based models in accuracy and efficiency. Beyond emulation, this paradigm shift has led to the development of hybrid climate models, where physics-based frameworks are augmented with trainable components, offering a new frontier in climate model design.
After a quick review of the progress and challenges in this rapidly evolving field, with a focus on ocean climate models, this webinar will explore the benefits of hybrid modelling, from parameterisations to bias correction, and provide insights into the practical design of trainable components. Additionally, it will address the technical complexities of integrating these components with existing model architectures.
Looking ahead, Julien Le Sommer will argue that current hybrid models foreshadow a new generation of AI-native climate models, powered by differentiable programming. These models promise seamless integration of trainable components and transformative capabilities, but they also pose significant challenges. This presentation will highlight the expected benefits of this emerging class of models and discuss the roadmap to harnessing their full potential in advancing climate modelling.
Autres Agenda TRACCS
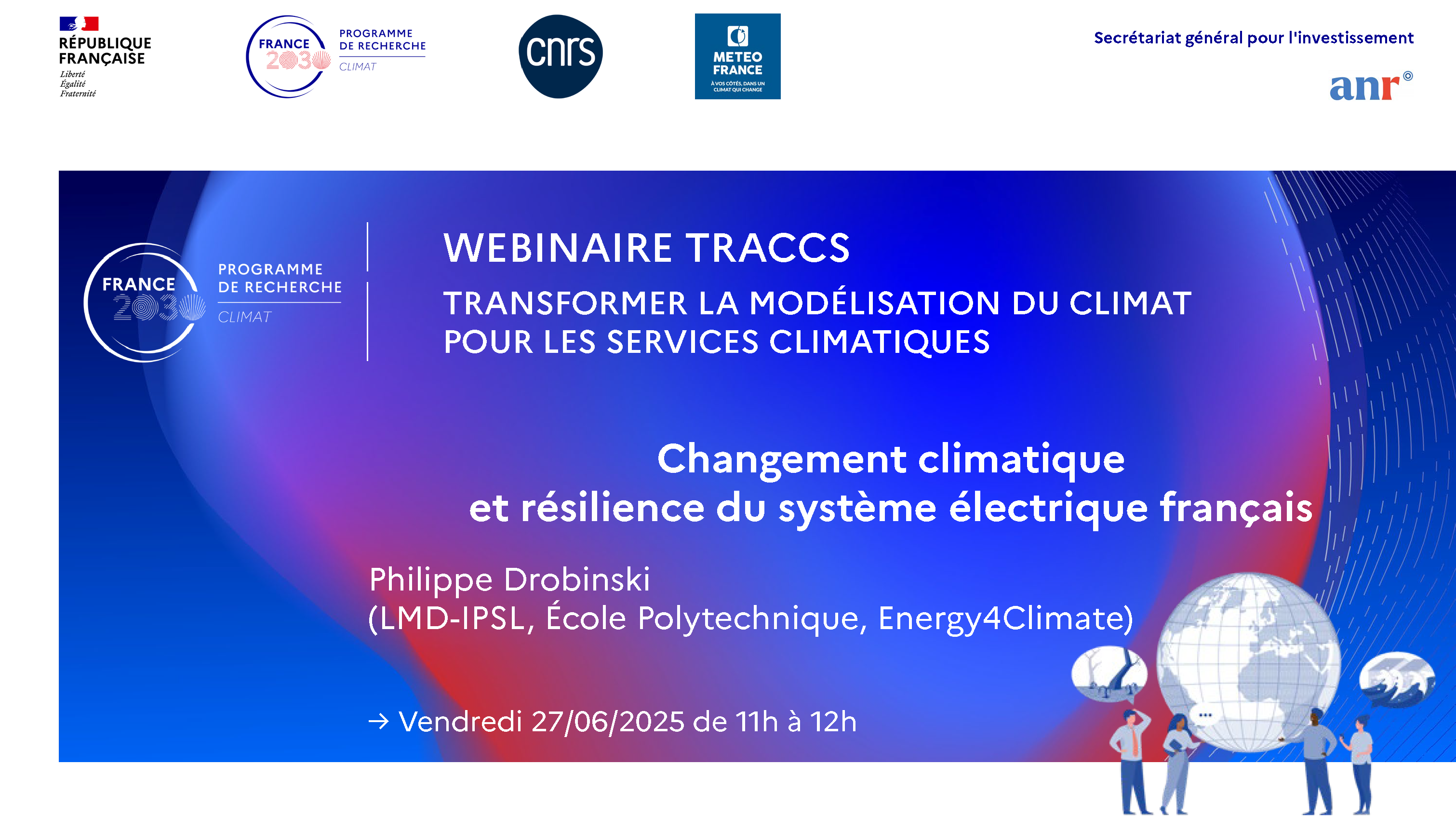
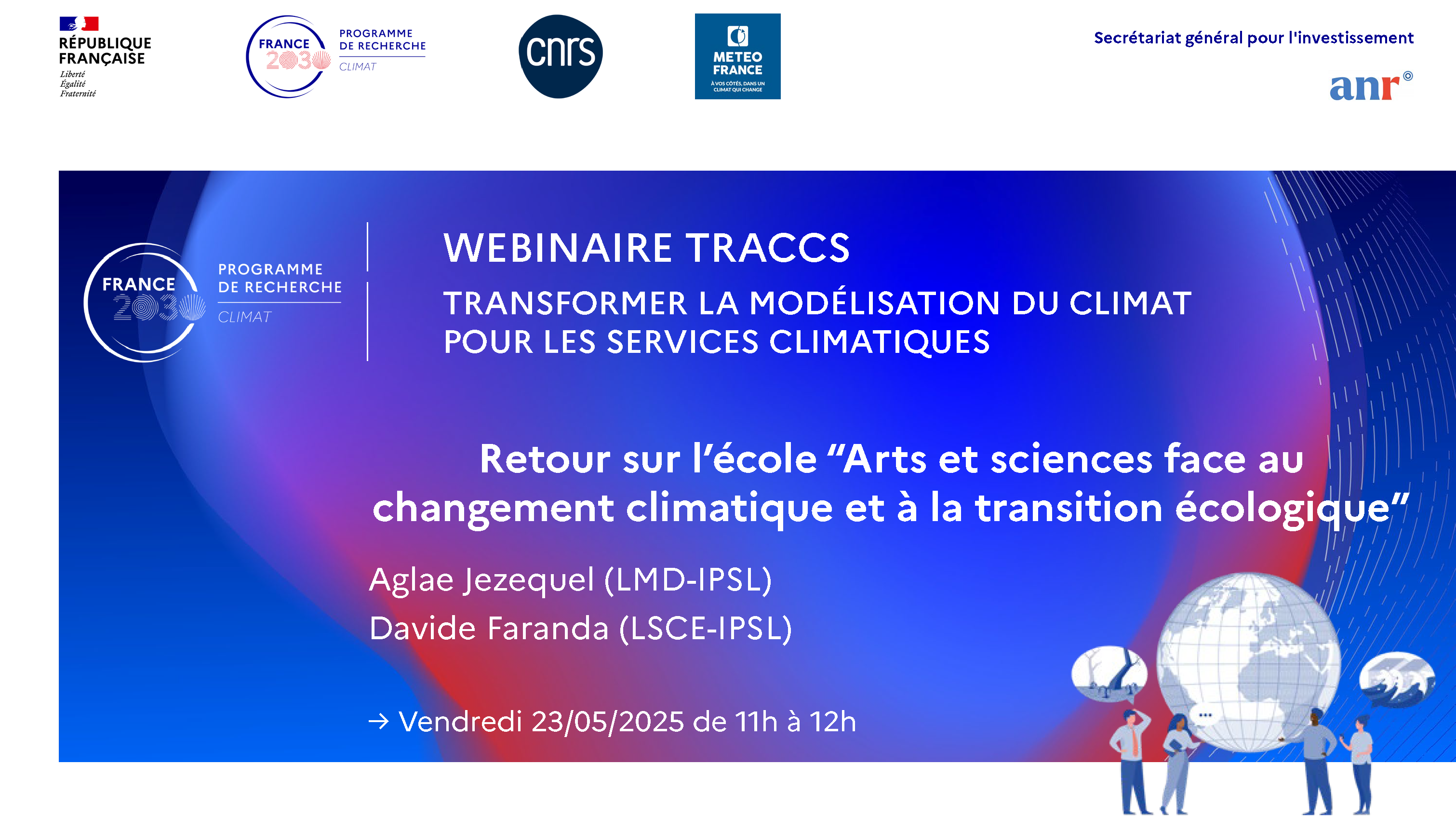
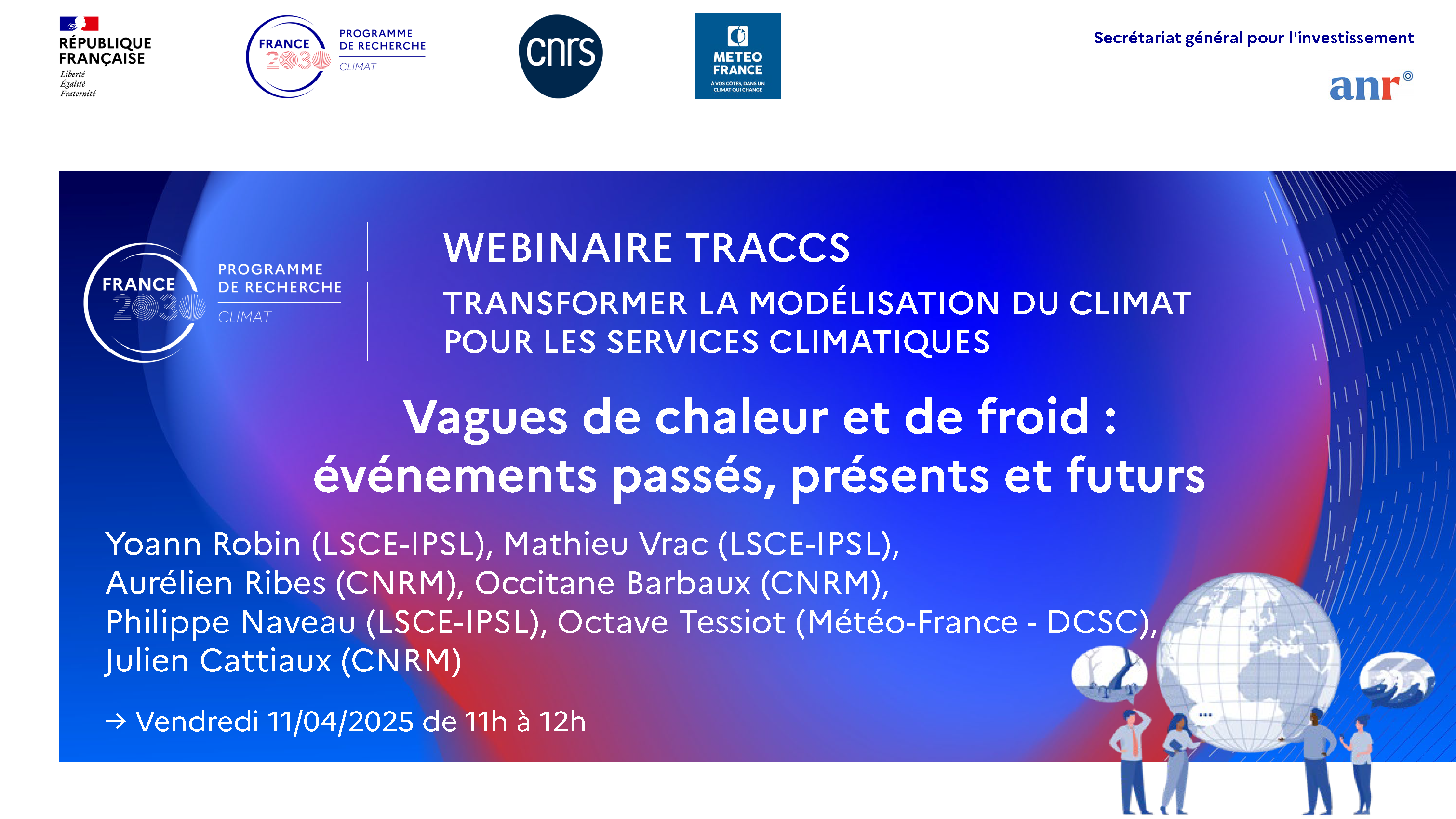